The “petabyte monster,” is quantitative trading’s infrastructure nightmare come to life.
Imagine over four decades of compounded data accumulation at a typical firm, routinely expanding into hundreds of petabytes, and still growing exponentially.
These datasets do not simply linger; they proliferate, exacerbated by expanding trading instruments, refined research methods, and the accelerating transition into AI-driven inference training.
As VAST’s Adam Jones points out, the COVID pandemic alone inflated trading data footprints roughly twofold in mere months, pushing the traditional parallel file-based HPC architectures used among quants to their limits.
This unprecedented avalanche of data, combined with increasingly outdated data movement tooling, has left trading firms grappling not just for competitive advantage but operational viability.
Trading infrastructures now sit at a critical crossroads: Already burdened under petabyte-scale problems, these systems are increasingly inadequate for the shift from traditional HPC methods (i.e. Monte Carlo simulations and massive quantitative batch processing) to more agile, AI-centric inference training models—ironically reliant upon the very data they struggle to manage.
What’s more, traditional HPC systems often necessitate tedious, latency-ridden data conversions, manually migrating vast datasets between separate silos (e.g., NFS to S3), squandering valuable processing cycles and impairing competitive agility.
To address this bottleneck, VAST Data presents a disaggregated shared-everything architecture that elegantly sidesteps traditional storage pitfalls.
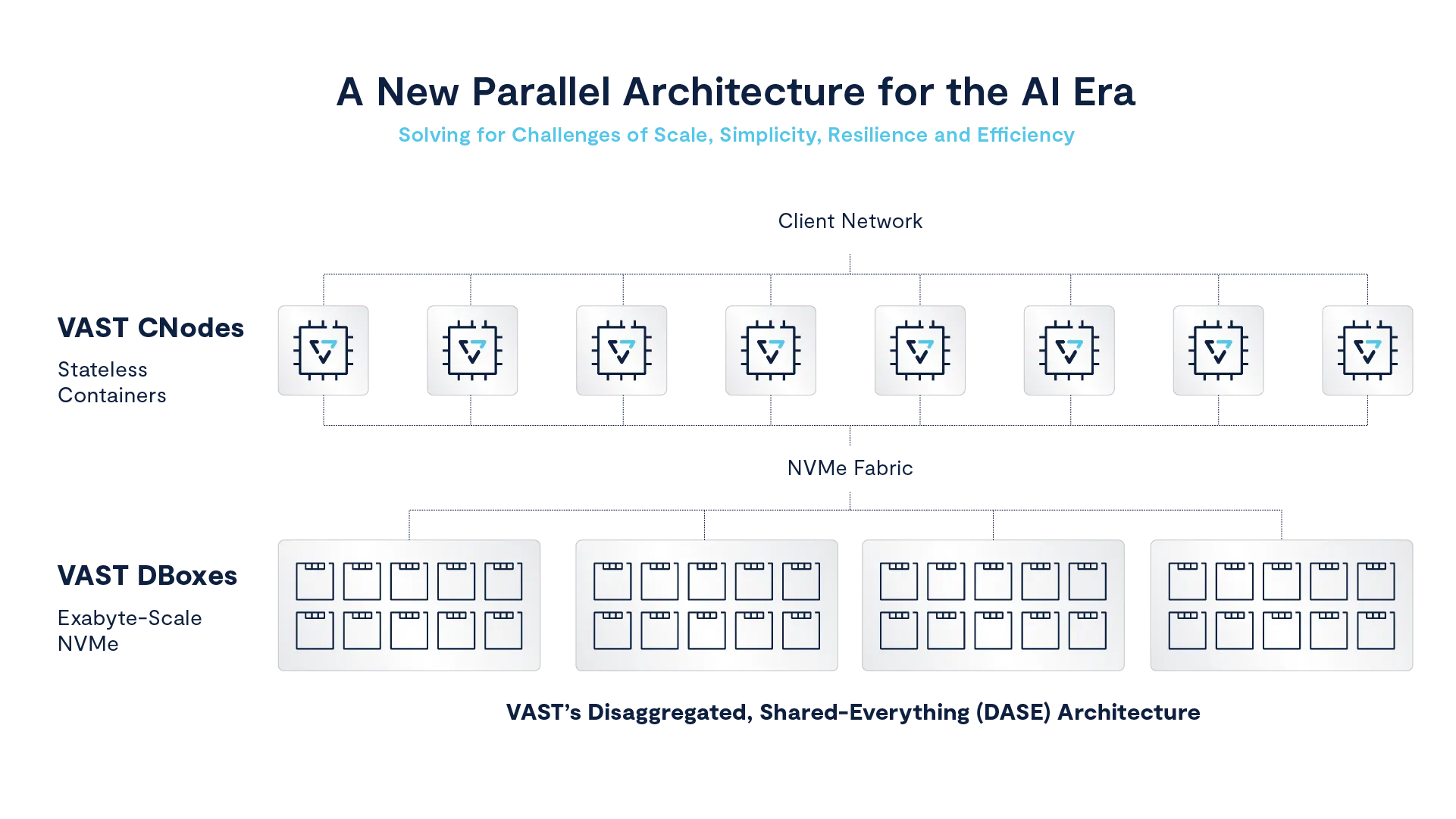
By distinctly separating storage performance from raw capacity, VAST’s platform transforms a once-clunky infrastructural divide into a frictionless operational continuum, effortlessly bridging legacy HPC workflows with contemporary AI applications.
Here’s how it works:
VAST deploys a dual-node architecture—stateless computational nodes (C nodes) and dedicated data-storage nodes (D nodes)—interconnected via a high-speed NVMe fabric. This separation allows computational horsepower and storage volume to scale independently, meaning firms can precisely calibrate their infrastructure without wasteful over-provisioning.
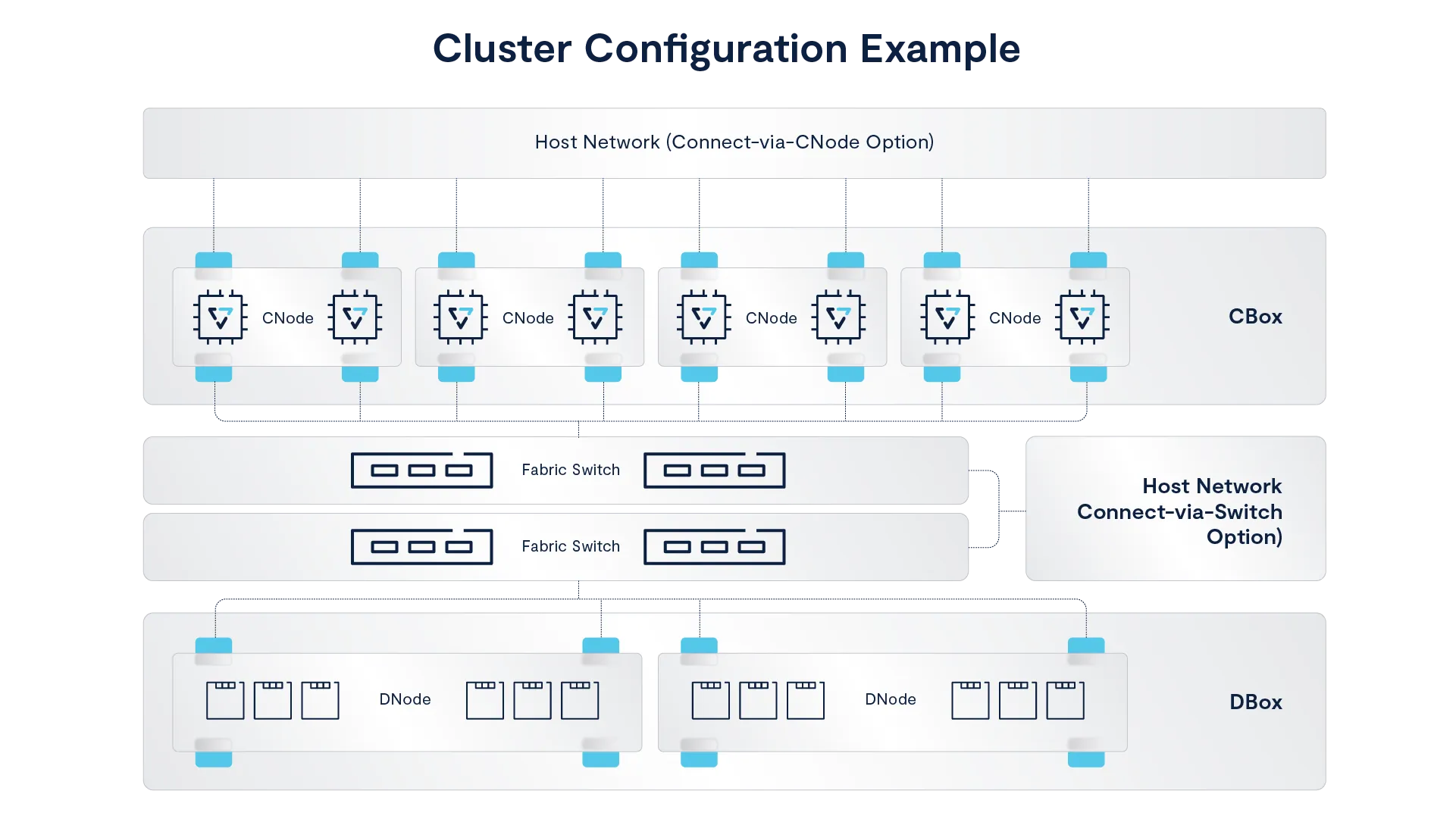
At its core lies VAST’s Element Store, a metadata-rich, protocol-agnostic data repository that natively supports NFS, SMB, S3, and block storage. Thus, data ingestion from HPC environments (think: massive quantitative batch processes) becomes immediately available for AI-driven inference tasks—without all that weighty dedupe or latency-inducing conversions.
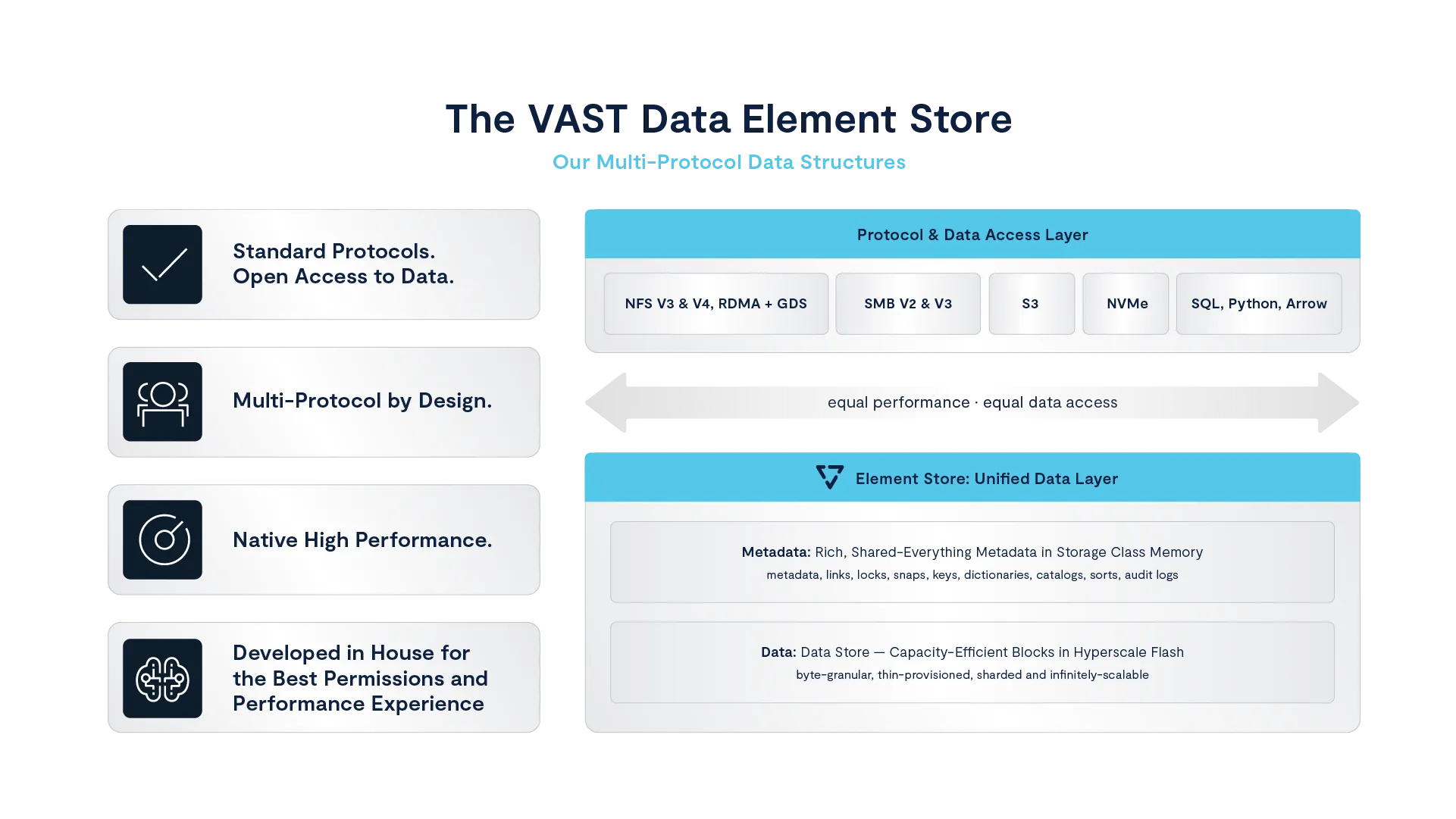
Even further, VAST’s “DataSpace” technology effortlessly unifies global data access, allowing geographically distributed trading teams real-time collaboration without the friction of manual transfers.
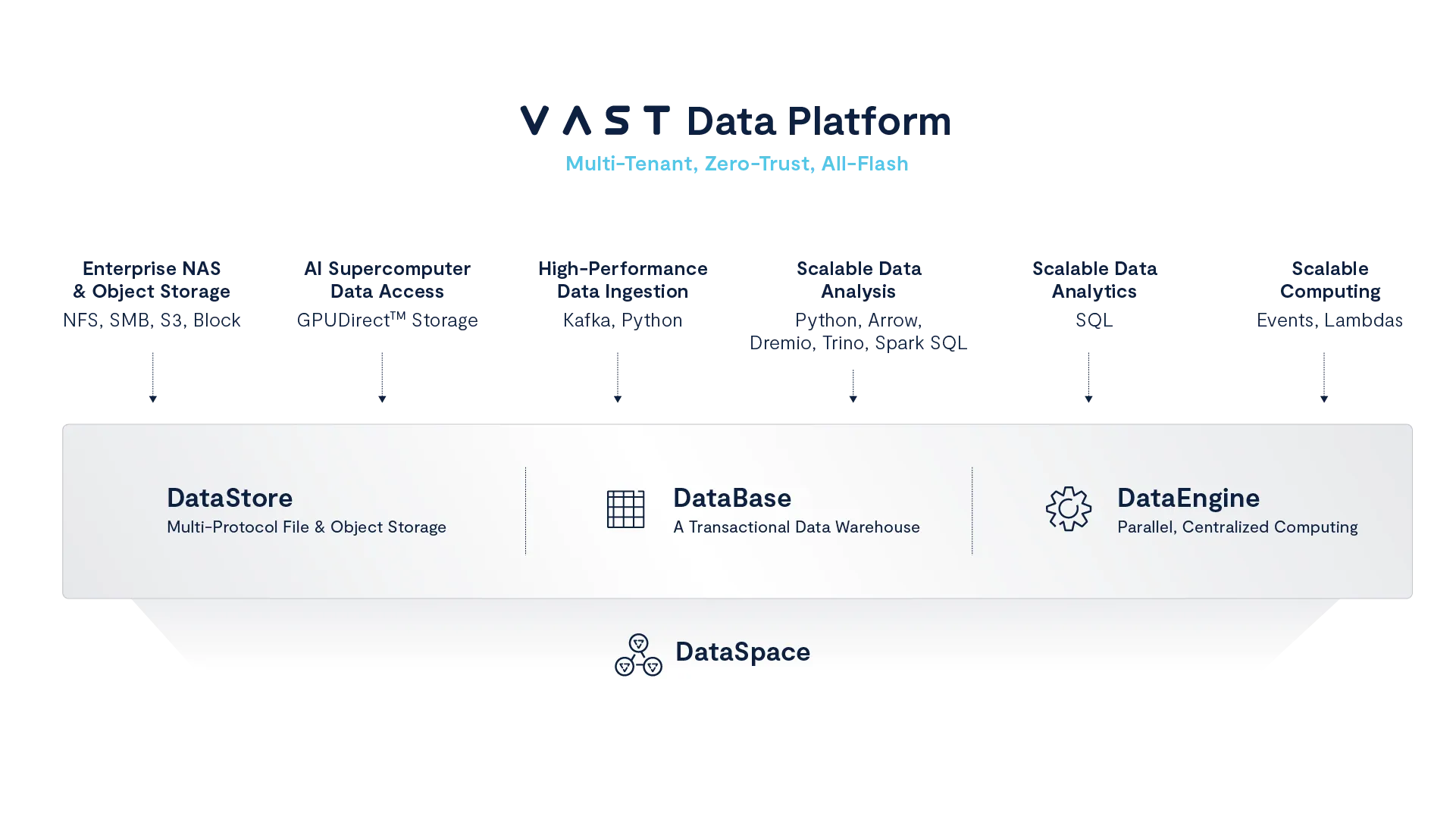
We see this work together with firms like Jump Trading, which leveraged VAST to nab flash-level performance at near HDD-level costs, significantly sharpening their competitive edge.
Similarly, trading house Squarepoint harnessed VAST’s unified namespace to enable seamless global collaboration, streamlining HPC and AI workflows across continents.
In short, VAST directly resolves the infrastructural nightmares haunting modern quantitative trading, reshaping massive complexity into graceful simplicity.
VAST doesn’t merely tame the petabyte monster—it politely convinces it to sit, stay, and perhaps fetch some AI insights.
By banishing manual interventions and streamlining once-clumsy HPC-to-AI transitions, VAST frees quantitative traders from wrestling with legacy technology, leaving them ample time to tackle more pressing concerns—like plotting world domination or figuring out why the coffee machine never works right during data migrations.