It’s not every day a hyperscale giant lifts the hood on its infrastructure.
Usually, us hacks are left to piece together whispers from conference talks, a patent filing here, a vendor leak there. But Meta’s recent paper doesn’t just crack a window–it practically throws open the doors, providing the most explicit architectural revelations yet about its internal AI network structure.
At the core, Meta’s architecture splits cleanly into two distinct connectivity domains, scale-up and scale-out, both of which are familiar terms but with some meaty new implications as described.
Let’s begin first with the scale-up domain, defined by tight intra-rack connections and staggering GPU density.
Here, direct-attached copper cables (DACs) link dozens of Blackwell GPUs, arranged in dense clusters within single racks—72 GPUs per rack, interconnected via nine NVLink5 switches at a blazing 200 Gbps lane rate.
As you can see below, each rack essentially becomes a self-contained unit that’s optimized to maximize bandwidth and trim latency, critical for syncing vast neural nets and their trillion-plus parameters.
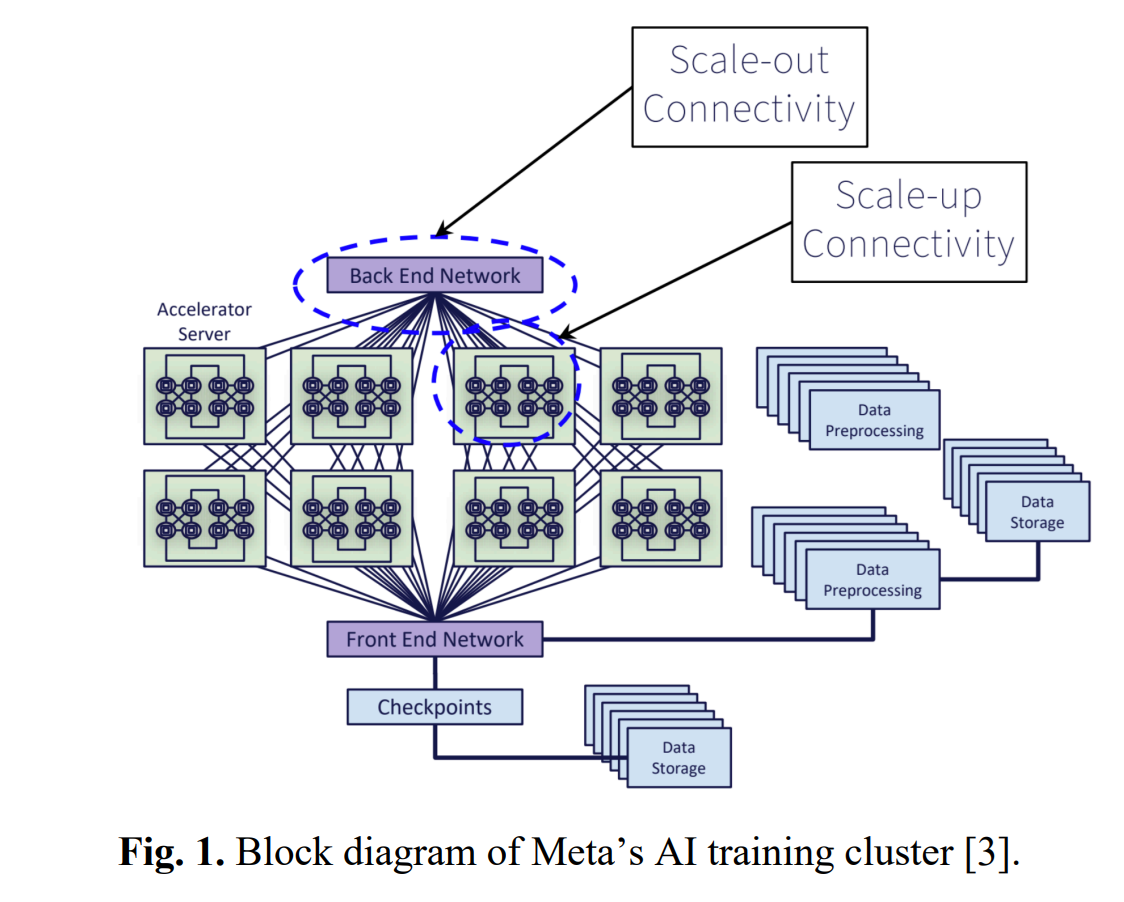
On one side, it captures dense, copper-linked GPU clusters within racks—the "scale-up domain." On the other, it shows these racks extending outward via optical fibers—the "scale-out domain"—illustrating clearly where copper's practical limits end and optics' potential begins.
Then, as shown above, there’s the scale-out domain, which spreads wide across multiple racks, interconnected through optical fibers.
Meta's approach here employs Remote Direct Memory Access (RDMA) over Converged Ethernet (RoCE). Pluggable optical modules bridge these separate islands, connecting racks via cluster-level switches, which further aggregate into multiple AI "zones."
This is hyperscale infrastructure laid bare, revealing something more akin to the architectural blueprints of a complex city rather than just a corporate datacenter.
But what’s most interesting, and perhaps most telling, are the specific challenges Meta admits to wrestling with.
Bandwidth density, power consumption, reliability, and latency aren’t theoretical issues anymore; they're real-world barriers Meta’s confronting right now.
Copper interconnects, while effective up to a point, quickly become limited by mortal physics at higher lane speeds, suffering severe signal lossage as they ramp toward the hundreds-of-GBs-per-second regime.
And as one might expect, the density of GPUs packed inside these racks pushes power and thermal envelopes right to their edge.
The reliability data Meta offers is equally striking. Think about this:
During a 54-day Llama 3 training run at a mind-boggling scale of 16,000 GPUs interruptions weren't rare hiccups but predictable, systemic events.
As the authors describe, each job interruption carries a steep cost, not just computationally, but operationally.
A single GPU node’s failure cascades into downtime and lengthy restart processes. Meta tackles this through meticulously optimized checkpointing strategies, which they detail as carefully timed state-saves designed to minimize training loss. But even with careful optimization, mean-time-between-failures (MTBF) requirements climb sharply into hundreds of thousands of hours per node as cluster scales increase.
As you might imagine, this puts optics under the spotlight.
The only feasible path forward at these scales are optical interconnects, which Meta argues must surpass demanding benchmarks–shoreline bandwidth density exceeding 1.2×N Tbps/mm beyond 2026, stringent MTBF thresholds, and drastically improved latency.
No sweat, right?
Meta is laying down the gauntlet: optics must mature rapidly to leap beyond these steep technical hurdles.
Further, they don’t mince words on what’s required for optical interconnects to escape the lab and into their datacenters: improved Forward Error Correction (FEC) strategies, crazy-low latency, and reliability metrics that would challenge even today’s most advanced hardware.
And by the real takeaway for the rest of us here–beyond the sheer depth of detail Meta provides–is why this matters even outside the walls of hyperscale giants.
Not every organization is building AI clusters with tens of thousands of GPUs, but many are scaling up to hundreds or thousands, encountering echoes of these same fundamental limitations.
GPU-to-GPU bandwidth constraints, copper interconnect losses, thermal density nightmares, and reliability concerns don’t disappear at smaller scales; they simply become somewhat less intense, but no less real.
In this sense, Meta’s paper isn't merely a rare glimpse inside hyperscaler racks; it's practically a roadmap for any organization charting their own AI infrastructure.
The explicit acknowledgment of these architectural challenges, combined with clearly defined technological goals for optics and the honest transparency about the difficulties of scale, provides invaluable guidance.
It’s a timely reminder that AI infrastructure at scale isn't just about assembling components but involves solving intertwined engineering puzzles around connectivity, efficiency, and reliability.
Ultimately, the importance of Meta's transparency isn't just about understanding their infrastructure, although that in itself is deeply instructive. Rather, it provides a glimpse into the future of AI infrastructure design, offering guidance to anyone tasked with building complex, GPU-intensive clusters.
This is how hyperscale architecture begins to reshape the broader landscape: not just through scale alone, but through the clarity of insight into its inherent limitations, pushing everyone else toward smarter, more resilient designs.